2/13/2025
Gradients Beats TogetherAI, Google to become the best 0-Click Training Platform in the World.
On-Chain
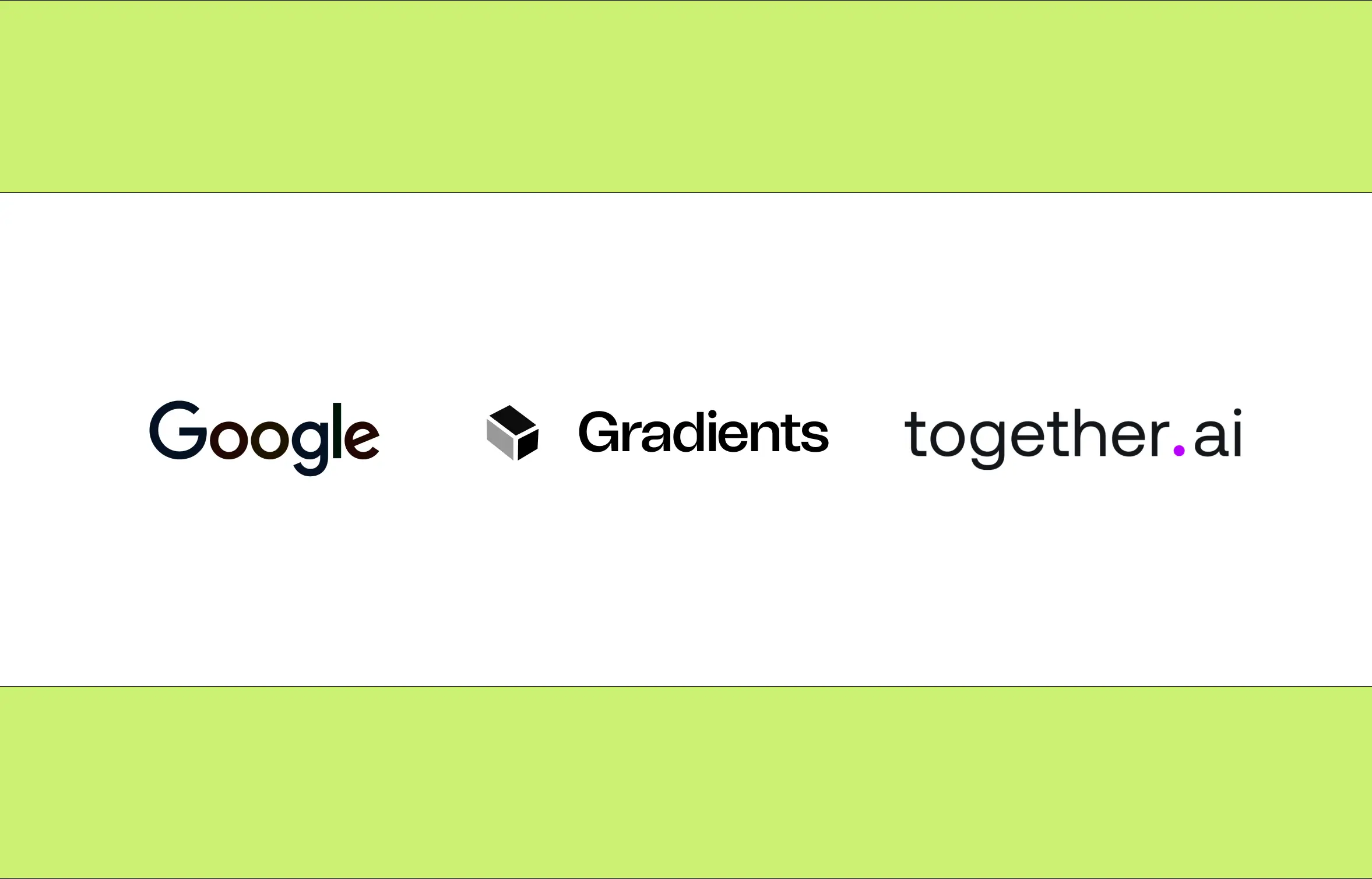
At Rayon Labs, we've been thinking about how we can push the boundaries of how we train large language models. The traditional approach has been dominated by deep pockets and niche expertise. But here's the real question: why should users themselves be stuck with all that complexity? Our mission is to let them focus on what truly matters—creating and innovating—while the technical heavy lifting is handled by a global network of specialised miners on Bittensor.
By transforming each training job into a decentralised micro-competition, we've shown that with a focus on user-friendliness customers can tap into expert-level model training without needing to swallow an AI textbook or know what terms like lora rank and weight decay are. The results have been incredible. Not only can a decentralised network refine existing models, it can also birth entirely new ones that stand shoulder-to-shoulder with industry favourites.
A Tale of Two Models
One of the bold promises of Bittensor is that a model born and pretrained on the network could rival the most respected instruction-tuned large language models out there. This week, we decided to use Gradients to put this to the test. We picked two 3B-parameter contenders:
- Llama 3.2: Meta's heavyweight, celebrated for its robust performance and backed by top-tier AI research.
- SN9: Our challenger, raised entirely on Bittensor's decentralised training network.
This wasn't just an exercise in model optimisation—it was a direct test of whether a Bittensor-native model could hold its own in the big leagues.
The Competition Unfolds
We kicked off a series of instruction-tuning competitions, inviting our network of miners to compete in optimising both models on a couple of popular and challenging datasets the LaMini-Instruction and the Google FLAN benchmarch. The outcomes were a huge win for decentralised AI on Bittensor.
First Round: LaMini-Instruction Benchmark
Second Round: Google FLAN Benchmark
Within a handful of competitions, both models improved dramatically—often by entire points in test loss. It is great to see, SN9 not only kept pace with Llama, but at times, it even outperformed it. More experimentation is undoubtably needed with a wider range of datasets and model families to realise the potential of this two subnet synergistic approch, but in the short time since launch we're very excited by this initial findings. There are tentative sproats of the great promise of decentralised AI being realised, a model pretrained on one subnet (9) and then fine-tuned on another (56) is capable of going head-to-head with an industry standard in foundational.
Raising the Stakes
Buoyed by these successes, we set out to compare our zero-click model training platform with some of the largest names in AI. In this one we take 8b Llama 3 and the biologic question instruct dataset from MlFoundations and the challenge is simple, which platform outputs the best fine-tuned model for a given computational time budget; Google, Together.AI or Gradients.
The findings speak volumes. With a test loss of 0.886, our decentralised system outpaced Google's 0.928 and Together.ai's 1.125. By giving miners the incentive to find the best hyperparameters and training strategies, we effectively harness a decentralised brain trust that delivers the best results for customers without the headache or expertise requirement.
PubMed QA: Beating SOTA with Decentralised Fine-Tuning
We didn't stop at general instruction tasks—we also turned our attention to a more specialised domain: PubMed QA, a challenging biomedical question-answering benchmark. Both the PubMed QA leaderboard and the Open Medical LLM Leaderboard rank models of varying sizes and architectures, making it a great testbed for progress.
Starting with a base Llama-3-8B, our standardised evaluation recorded a baseline accuracy of 78%. After fine-tuning with Gradients, we achieved an impressive 80.2%—not only outperforming the base model but also eclipsing the highest published accuracy on the leaderboard. This milestone marks the first SOTA model trained on Gradients.
The Power of the Crowd
These weren't isolated wins. In a matter of weeks, our platform facilitated more than 4,000 training jobs, each yielding 6-8 high-quality submissions. Think about that for a moment: we're dealing with models totalling 120,000 billion parameters, trained on over 5,400GB of text data. All in a fraction of the time and cost demanded by traditional methods.
This success hinges on a core principle: every training job becomes a fierce competition, prompting miners to bring their A-game. In return, they earn rewards, and users get fine-tuned models that would normally require a small army of in-house experts.
The Future is Decentralised
We've shown that decentralised networks aren't just good at training—they're capable of producing formidable models. Our next step is to expand this approach beyond text, with vision models waiting in the wings.
As we integrate new features, we're staying true to what brought us here in the first place: a competitive, decentralised model that democratises expertise. Llama vs. SN9 was just the beginning, and we're excited to see how the power of the crowd continues to reshape AI development.
We believe a future where AI expertise is accessible to everyone isn't just possible—it's inevitable. And we can't wait to be a part of that journey.